Knowledge graphs have traditionally been pivotal in structuring and reasoning about complex information using textual data, depicting entities and their relationships in a graph format. However, with the exponential growth and diversity of digital content, there arises a need for more inclusive representations. Enter multimodal knowledge graphs, which go beyond text-only formats by incorporating data from various modalities such as images, videos, and audio. This integration aims to provide a more holistic view of entities and their interactions, enhancing the capabilities of knowledge-driven systems across diverse domains.
The Benefits of Multimodal Knowledge Graphs
These graphs have several benefits including:
- Richer Representation of Entities: Unlike traditional knowledge graphs limited to textual descriptions, multimodal knowledge graphs integrate visual, auditory, and other data types. For instance, a knowledge graph about artworks can include not only textual metadata but also visual features of paintings, enabling deeper insights and more nuanced analysis.
- Enhanced Contextual Understanding: By combining multiple modalities, multimodal knowledge graphs facilitate a more comprehensive understanding of entities. For example, in a healthcare scenario, integrating patient records with medical images can provide a clearer picture of a patient’s condition than text alone.
- Improved Reasoning Capabilities: Multimodal knowledge graphs enable more sophisticated reasoning by leveraging multimodal data. This is particularly valuable in applications requiring complex decision-making, such as personalized recommendation systems or diagnostic tools.
Challenges in Constructing Multimodal Knowledge Graphs
Here are the top challenges faced in constructing these graphs:
- Data Heterogeneity: Integrating data from diverse modalities poses challenges due to differences in structure, format, and semantics. Aligning textual descriptions with visual or auditory data requires advanced techniques for data fusion and alignment.
- Multimodal Entity Linking: Identifying and linking entities consistently across different modalities can be difficult. For example, linking a person’s name mentioned in text with their image in a photograph requires robust entity linking algorithms that can handle multimodal inputs.
- Multimodal Relation Extraction: Extracting meaningful relationships between entities based on multimodal data is complex. Techniques must account for how different modalities interact and contribute to understanding relationships within the knowledge graph.
- Scalability and Efficiency: As multimodal knowledge graph grows larger in scale and complexity, maintaining efficiency in storage, processing, and querying becomes crucial. This challenge is exacerbated when dealing with vast amounts of multimodal data.
Advances in Multimodal Knowledge Graph Construction
Researchers have made significant strides in overcoming these challenges, developing innovative techniques and frameworks:
- Multimodal Entity Linking: Advanced deep learning models, such as transformer-based architectures, have shown promise in aligning entities across textual and visual modalities. These models can learn to link entities based on both textual context and visual features.
- Multimodal Relation Extraction: Novel neural network architectures have been proposed to capture and model interactions between different modalities effectively. These models can extract complex relationships by jointly considering textual, visual, and other modal data representations.
- Multimodal Knowledge Graph Embedding: Techniques for embedding these graphs into low-dimensional vector spaces have been developed. These embeddings facilitate efficient storage and enable downstream tasks such as knowledge graph completion and inference.
- Multimodal Reasoning: Techniques like multimodal attention mechanisms and reasoning frameworks have been explored to leverage the rich information in these graphs. These advancements enhance the ability of systems to answer complex queries and support decision-making processes.
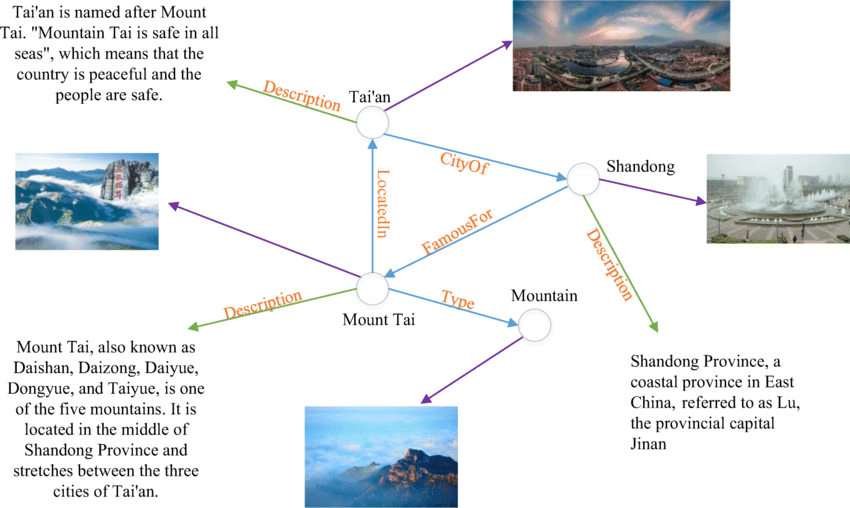
A Simple Example of Multimodal Knowledge Graph; Source: ResearchGate
Key Application Areas
MMKGs hold immense potential across various domains:
- Healthcare: Integrating patient records with medical images and clinical notes can improve diagnostic accuracy and treatment planning.
- Education: Enhancing educational platforms with multimodal knowledge graphs can personalize learning experiences based on diverse modalities of student interaction and performance data.
- Cultural Heritage: Multimodal knowledge graphs can enrich cultural heritage preservation efforts by integrating textual descriptions, images, and historical records.
- E-commerce: Recommender systems can benefit from multimodal knowledge graphs by incorporating user preferences expressed through text, images of past purchases, and reviews.
What is Next?
While current advancements are promising, several research directions remain:
- Benchmarking Multimodal Knowledge Graphs: Establishing standardized benchmarks and evaluation protocols will facilitate fair comparisons between different multimodal knowledge graph construction methods.
- Dynamic Multimodal Knowledge Graphs: Exploring how multimodal knowledge graphs can evolve over time with new data inputs and changing contexts is critical for real-world applications.
- Explainability and Trust: Developing methods to explain multimodal knowledge graph-driven decisions and predictions will enhance user trust and adoption in diverse applications.
Final Words
Multimodal knowledge graphs represent a significant leap in the evolution of knowledge representation and reasoning systems. By integrating textual, visual, and other data modalities, multimodal knowledge graphs enable richer, more nuanced understanding of entities and their relationships. Despite challenges in data integration and scalability, recent advancements in deep learning and multimodal fusion techniques have paved the way for practical applications in healthcare, education, and beyond. As research continues to progress, the future of multimodal knowledge graphs holds promise in revolutionizing how we interact with and harness complex real-world knowledge.