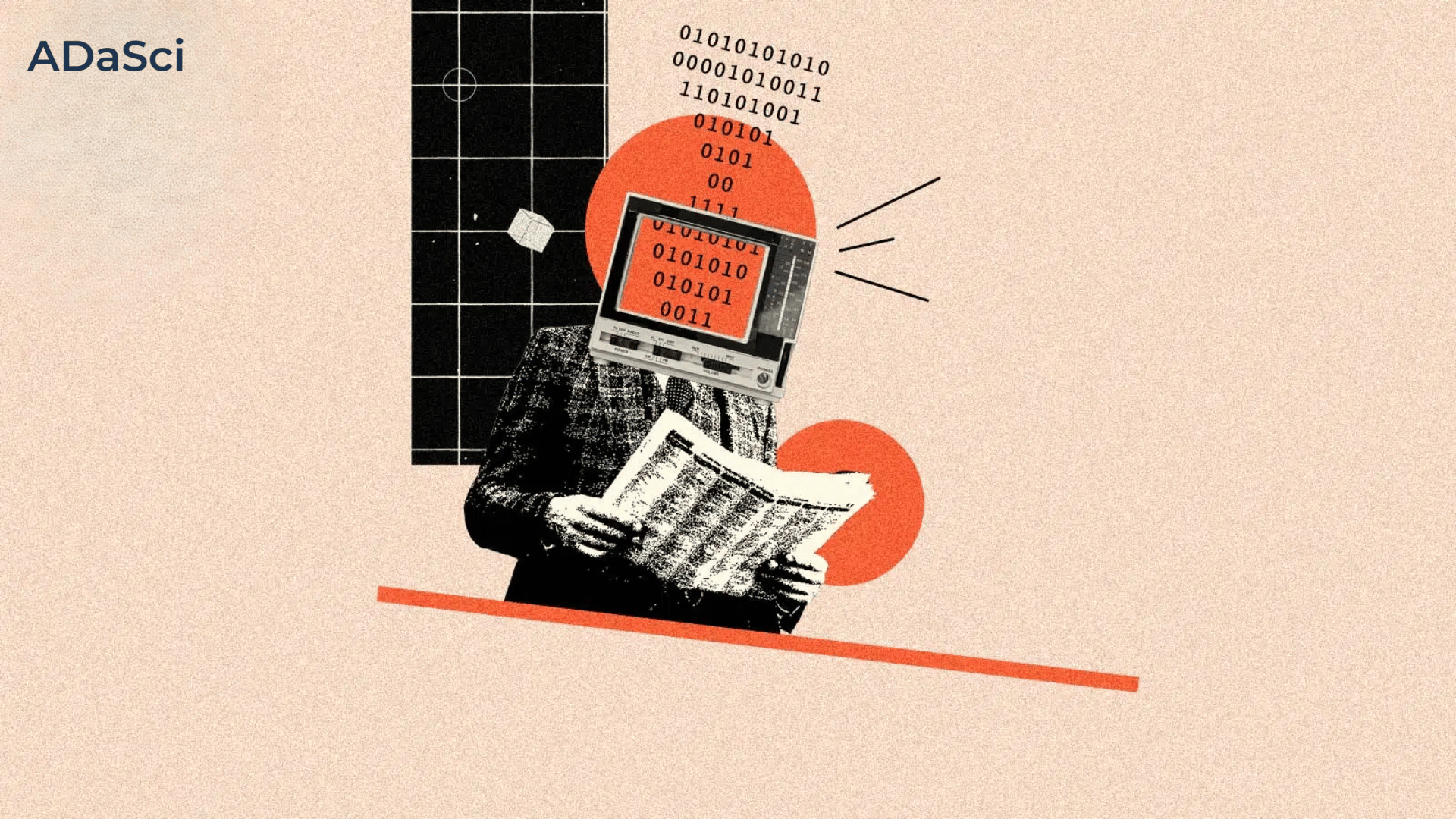
Mastering Data Compression with LLMs via LMCompress
LMCompress uses large language models to achieve state of the art, lossless compression across text,
LMCompress uses large language models to achieve state of the art, lossless compression across text,
AlphaEvolve by DeepMind evolves and optimizes code using LLMs and evolutionary algorithms, enabling breakthroughs in
J1 by Meta AI is a reasoning-focused LLM judge trained with synthetic data and verifiable
The highest distinction in the data science profession. Not just earn a charter, but use it as a designation.
OpenAI’s Agents SDK enables efficient multi-agent workflows with context, tools, handoffs, and monitoring.
Portkey enables observability and tracing in multi-modal, multi-agent systems for enhanced understanding and development.
PydanticAI Agents leverage Pydantic’s validation to build reliable, type-safe AI decision-making systems.
Nexus is a lightweight Python framework for building scalable, reusable LLM-based multi-agent systems.
Mixture-of-Mamba enhances State Space Models for efficient multi-modal data processing across text, images, and speech.
DeepSearch revolutionizes question-answering in LLMs, enhancing precision, completeness, and efficiency in information retrieval.
Unstract automates document processing with AI, reducing manual effort, errors, and costs.
DeepSeek’s R1 model revolutionizes AI reasoning, balancing reinforcement learning with structured training techniques.
LLM systems gain powerful monitoring and optimisation capabilities through Literal AI’s comprehensive observability and evaluation
ElasticSearch’s vector search capabilities enable intelligent, context-aware applications through AI-powered semantic understanding.