Tokenization has long been the cornerstone of large language models (LLMs), but it introduces limitations in efficiency, robustness, and multilingual equity. Enter the Byte Latent Transformer (BLT), a revolutionary tokenizer-free architecture that learns directly from raw byte data. BLT matches token-based LLMs in performance while surpassing them in inference efficiency and robustness. In this article, we explore how BLT achieves this breakthrough, its key features, implementation details, and practical applications.
Table of Content
- Introduction to BLT
- Key Features of BLT
- Key Implementation Steps
- Practical Use Cases
- Technical Deep Dive
Introduction to BLT
The Byte Latent Transformer is a novel architecture that dynamically groups bytes into patches, enabling efficient computation at scale. Unlike token-based models, BLT does not rely on fixed vocabularies, mitigating issues like input noise sensitivity and language biases. Its design introduces a new scaling axis—simultaneously increasing patch and model size—without additional inference costs.
Why Does BLT Matters?
Traditional LLMs allocate equal compute to all tokens, leading to inefficiencies. BLT’s dynamic patching allows compute allocation based on data complexity, resulting in a more robust model. This approach is particularly valuable in tasks requiring long-tail generalisation and noise handling.
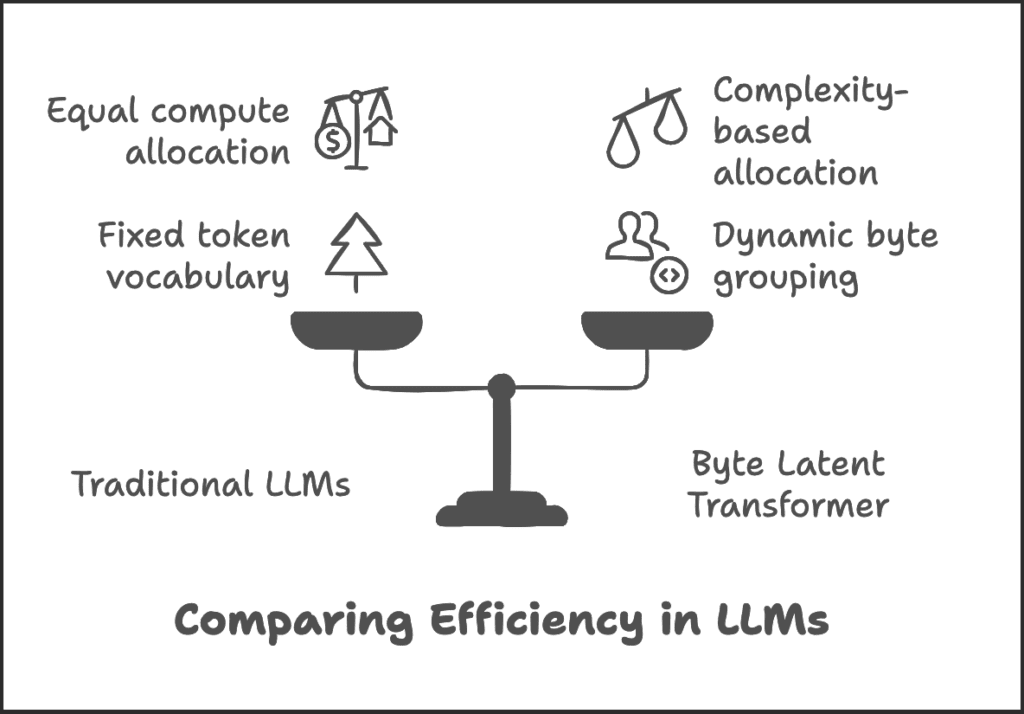
Comparing Efficiency in LLMs
Key Features of BLT
Dynamic Byte Patching
BLT segments raw bytes into entropy-based patches, ensuring computational resources are allocated where needed most. This approach reduces inference costs significantly compared to traditional token-based models.
Token-Free Design
BLT’s patching eliminates the need for tokenization, allowing it to directly model byte-level data. This results in improved robustness against noisy inputs and better handling of multilingual and domain-specific data.
Scalable Architecture
BLT demonstrates superior scaling properties, maintaining performance parity with state-of-the-art token-based models like LLaMA 3 while offering up to 50% inference efficiency improvements.
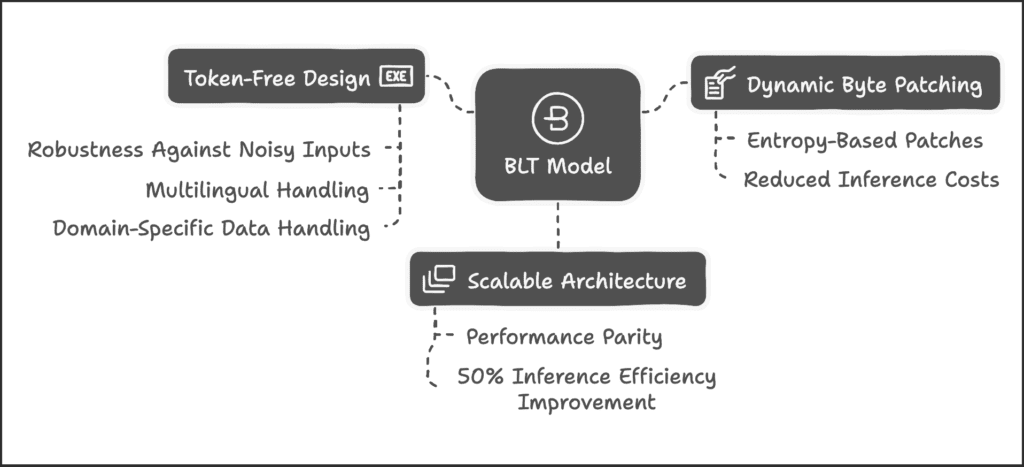
Key Features of BLT
Key Implementation Steps
Data Preparation:
- Use raw byte streams instead of tokenized data.
- Ensure entropy-based patching during data preprocessing.
Model Architecture:
- Integrate a Local Encoder, a Latent Transformer, and a Local Decoder.
- Employ cross-attention mechanisms for dynamic byte grouping.
Training Configuration:
- Utilize a FLOP-controlled training regime.
- Experiment with patch sizes (e.g., 6 or 8 bytes) to optimize performance and efficiency.
Practical Use Cases
BLT’s flexibility and efficiency open up numerous applications:
- Multilingual NLP: Robust handling of low-resource languages without biases from fixed token vocabularies.
- Code Generation: Improved modeling of programming languages, as seen in tasks like HumanEval and MBPP.
- Robust Text Processing: Enhanced performance in noisy data environments, such as social media text analysis.
Technical Deep Dive
Entropy-Based Patching:
- Segment data dynamically using next-byte entropy predictions.
- High-entropy regions receive more computational focus.
Model Components:
- Local Encoder: Converts byte sequences into patches using lightweight transformers.
- Latent Transformer: Processes patch representations with global attention.
- Local Decoder: Reconstructs byte sequences from patch representations.
Efficiency Optimization:
- Larger patch sizes reduce inference steps, reallocating compute to expand the global transformer.
- Utilize n-gram hash embeddings to enhance byte representation.
Best Practices
- Use entropy thresholds to dynamically adjust patch sizes.
- Pair cross-attention mechanisms with pooling-based query initialization for better patch representation.
- Leverage FLOP-controlled scaling studies to optimize training and inference costs.
Final Thoughts
The Byte Latent Transformer redefines efficiency and scalability. Its innovative approach to dynamic byte patching and token-free modeling makes it a game-changer, especially for applications demanding robustness and low inference costs. As BLT continues to evolve, it promises to unlock new possibilities for large-scale language models.
References
- Research Paper
- Official Codebase: GitHub