Artificial intelligence is entering a transformative phase. Moving beyond single-task tools, we’re now seeing the rise of agentic AI—systems capable of autonomous planning, decision-making, and execution. At the same time, emerging interoperability protocols like MCP (Model-Context Protocol) and A2A (Agent-to-Agent) Protocol are enabling seamless collaboration between agents across systems. And with the rise of Vibe coding, an event-driven, reactive development paradigm, developers are building agile, real-time pipelines that respond to user inputs, data changes, and inter-agent triggers.
For learning and development (L&D) leaders, these shifts are more than technical milestones—they’re strategic signals. Understanding these trends, evaluating readiness, and preparing a robust talent transformation roadmap is now essential.
1. Key Trends Shaping the AI Landscape
Understanding the forces driving the next generation of AI systems is essential for building future-ready strategies and talent pipelines.
1.1 Agentic AI: Beyond Co-Pilots to Autopilots
Agentic AI goes a step beyond generative assistants. These systems can autonomously interact with environments, other agents, and APIs to complete complex tasks with little to no human intervention. From multi-agent systems handling dynamic customer support to research automation and logistics optimization, agentic frameworks are being embedded into enterprise workflows. Current deployments operate at semi-autonomous levels (Levels 2–4), but we’re rapidly approaching fully autonomous Level 5 scenarios where AI agents are not just assisting but orchestrating entire processes.
1.2 Interoperability Through MCP & A2A
The development of standard communication protocols is a crucial backbone for AI at scale. MCP enables structured, reliable messaging between components in distributed systems, allowing agents to operate across platforms with reduced friction. A2A protocols build on this by enabling peer-to-peer discovery, negotiation, and task coordination between agents. These protocols are foundational for modular, scalable AI ecosystems—especially in enterprise environments with legacy systems, cloud services, and domain-specific tools that must work together seamlessly.
Join our upcoming hands-on workshop:
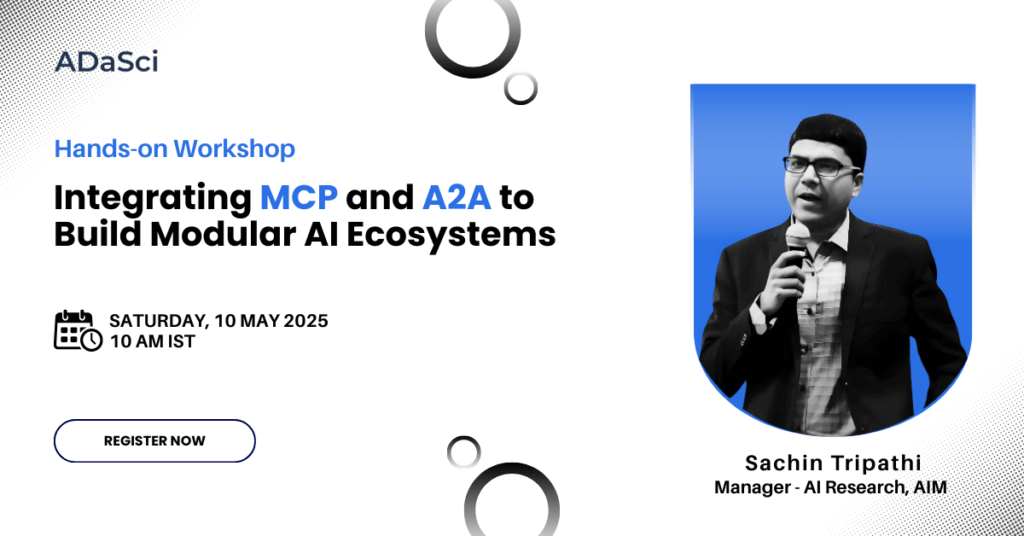
1.3 Vibe Coding and Event-Driven Workflows
Vibe coding represents a new model for building intelligent systems—reactive, event-driven, and inherently agent-aware. Using domain-specific languages like VibeScript or visual tools like VibeFlow, developers can define how agents respond to events (data updates, user actions, or external triggers). These frameworks enable fast iteration, low-code accessibility, and native support for distributed systems, making them ideal for dynamic use cases like personalized content delivery, automated reporting, or live inventory tracking.
2. Assessing Organizational Readiness
Before diving into implementation, it’s essential to take a structured look at your organization’s AI readiness. Rather than depending on any single commercial model, combine core principles from established AI readiness frameworks. Start by evaluating data maturity, infrastructure, and governance, ensuring you have the necessary quality, accessibility, and security protocols in place. Assess the skill levels of both technical and non-technical teams, as well as your organization’s ability to adapt culturally and operationally to autonomous AI systems.
Look into existing automation initiatives and pilot projects, identifying both the barriers and accelerators. Finally, build a phased roadmap that includes risk assessment, ethical guidelines, and measurable outcomes. This holistic view will help L&D leaders identify where transformation is required—not just in technology, but in mindset and workflow integration.
3. Preparing Your Talent for the Agentic Era
Equipping your workforce with the right skills, tools, and mindset is crucial to fully leverage the potential of agentic and interoperable AI systems.
3.1 Technical Talent Development
For software engineers, data scientists, ML engineers, and AI architects, specialized upskilling paths are critical. Programs offered by ADaSci (Association of Data Scientists) provide targeted certifications aligned with the evolving AI landscape. These include:
- Certified Agentic AI Architect – Focuses on designing and deploying autonomous agent ecosystems using multi-agent orchestration frameworks, communication protocols, and ethical alignment.
- Certified Generative AI Engineer – Covers generative modeling, prompt engineering, fine-tuning large language models, and production-scale deployments.
- Certified Data Engineer – Equips professionals with skills in building data infrastructure and pipelines necessary for real-time AI systems.
To complement these programs, enterprises should conduct hands-on workshops in agentic AI stacks like LangChain, Microsoft AutoGen, and MCP/A2A implementations. Hosting Agent Hackathons—where cross-functional teams build AI workflows using Vibe coding tools—can rapidly accelerate learning and innovation while fostering collaboration.
3.2 Non-Technical & Hybrid Roles
For customer service teams, operations staff, and business managers, it’s essential to demystify AI and build confidence in working alongside autonomous systems. Instead of relying solely on theory-based learning, organizations should prioritize simulation-based, gamified programs. One strong example is Datalyze by MachineHack, which provides scenario-driven, role-based training where users interact with AI systems in virtual environments. These simulations allow users to experience real-time decision-making, reinforce their understanding of AI-driven processes, and develop trust in co-working with intelligent systems.
To institutionalize continuous growth, organizations can create internal “AI Fluency Tracks” tailored to different functions—marketing, HR, finance—covering how agentic systems influence workflows, compliance, and performance KPIs. These tracks should be supplemented with AI literacy micro-courses, short, consumable modules explaining foundational concepts such as model bias, explainability, and human-in-the-loop governance.
3.3 Building Long-Term Capability
Establishing a culture of continuous learning is as important as individual training programs. Organizations should:
- Launch AI ambassador networks, where trained champions in each business unit mentor peers and share emerging best practices.
- Integrate AI talent forecasts into workforce planning to proactively identify new roles and skills needed as automation evolves.
- Offer career progression incentives for those earning certifications like those from ADaSci, giving employees a clear path to AI-focused leadership roles.
These initiatives ensure that AI learning isn’t a one-time event but an embedded element of professional development.
Final Words
The convergence of agentic AI, interoperability protocols like MCP and A2A, and the rise of Vibe coding represent a new chapter in AI adoption. For L&D leaders, the challenge isn’t simply about training a few technical experts—it’s about preparing entire organizations to function alongside intelligent systems. By staying attuned to emerging trends, rigorously evaluating readiness, and launching targeted, role-specific upskilling programs (such as those offered by ADaSci and gamified tools like Datalyze), organizations can position themselves to not just adapt, but lead in the agentic AI future.